Our paper was just published online in the journal Computers, Environment and Urban Systems: Evaluating Package Delivery Crowdsourcing using Location Traces in Different Population Densities by Ayelet Arditi and Eran Toch. In the paper, we use massive data about people’s movements to simulate and analyze parcel delivery services. We would love it if people would download it, read it, and send us feedback!
In the paper, we have looked at crowdsourcing delivery services for parcel delivery (imagine DoorDash, Uber, Postmates, etc) and wondered how these services can work in different locales (densely urban areas, suburbs, rural areas, etc), with different architectures, and under different demand models. We were particularly interested in architecture in which parcels can be left in lockers, and to see if they can help make the system more efficient.
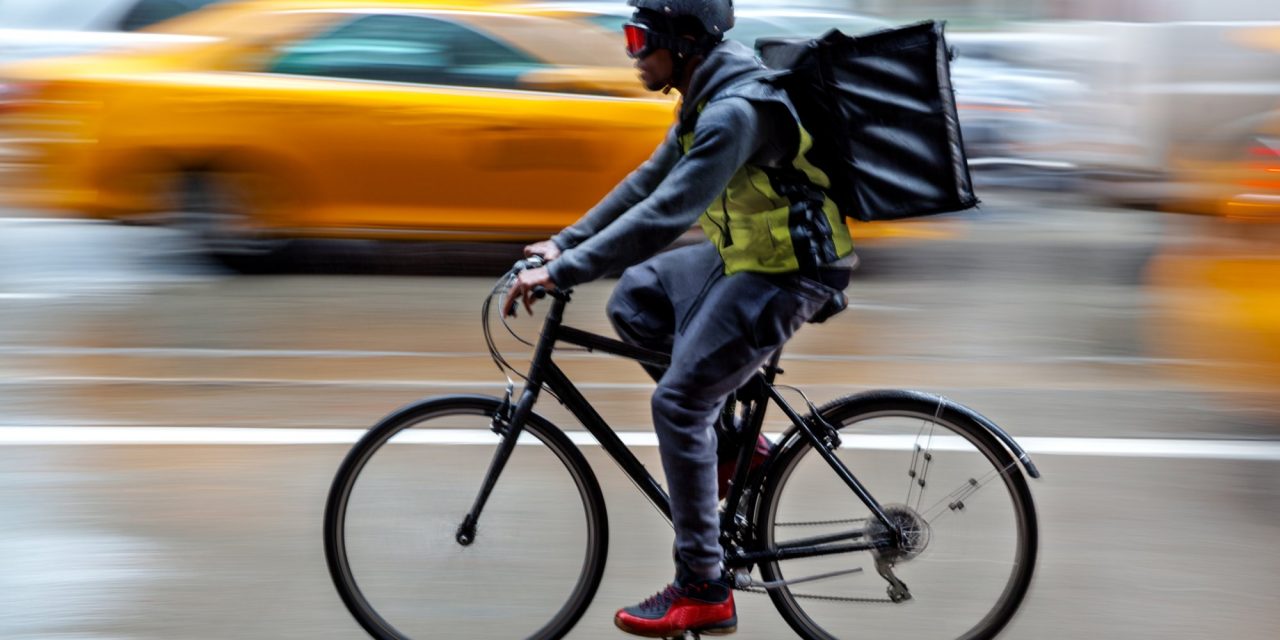
Crowdsource parcel delivery
So how can we evaluate systems without actually having an actual system running, we have used aggregative location traces of 1.8 million Israelis to simulate crowdsourced package delivery systems. We have analyzed the performance of crowdsourcing architectures under different participation rates and population densities.
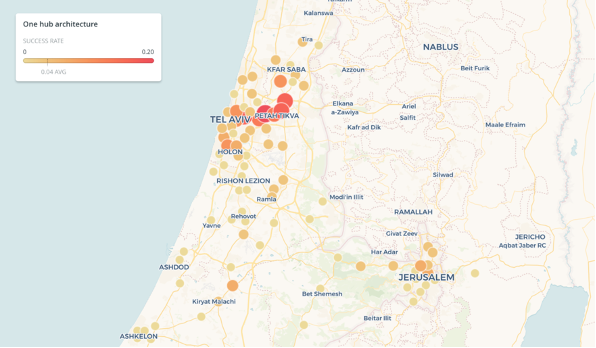
Success rates of networks with one hub stop point with a 10% participation rate. The color and size of the stop points encode the success rate of a network in which the stop point serves as a hub stop point
Our results show that areas with low population density are harder to serve, and require a larger number of locker sites for a similar level of service. Performance drops to unattainable levels in areas with low population density in existing transportation volume conditions Our understanding is that without subsidizing crowdsourcing services in rural areas, it is hard to imagine how they can work.
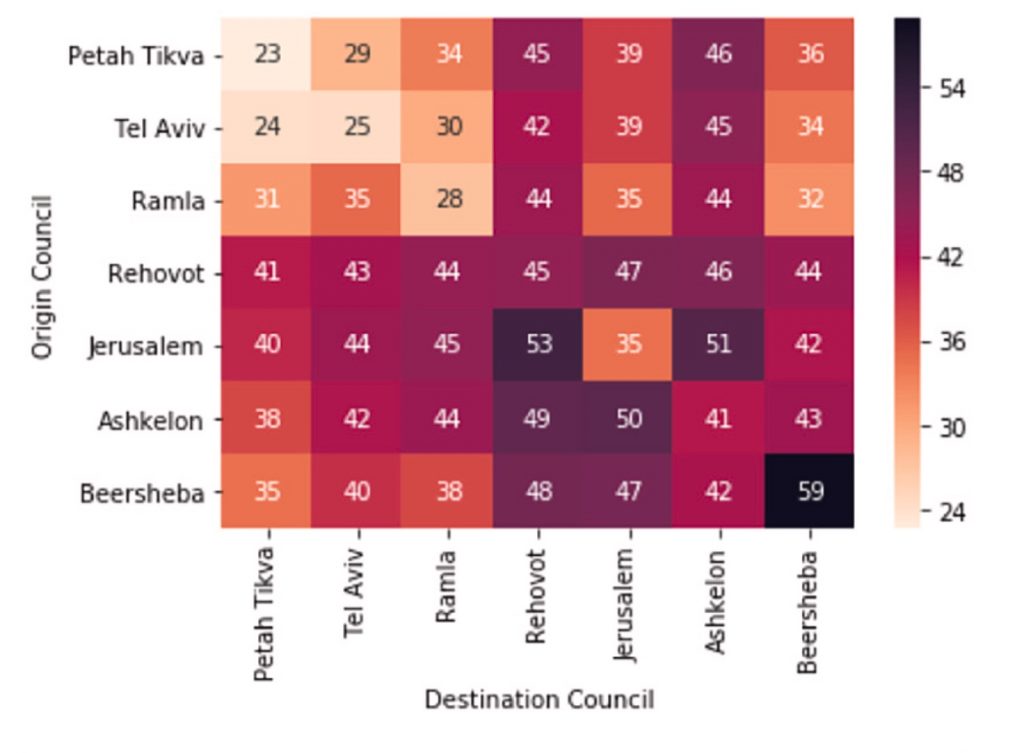
The delivery duration (in hours) between and within areas in Israel with intermediate lockers
We are also very excited about using our framework to simulate “imaginary” services, such as autonomous ride-sharing cars, delivery services, or mobile sensing systems, and use data about people’s movements to design and “run” them in different areas and under different conditions. Imagine having something like that before you raise money for your next startup.
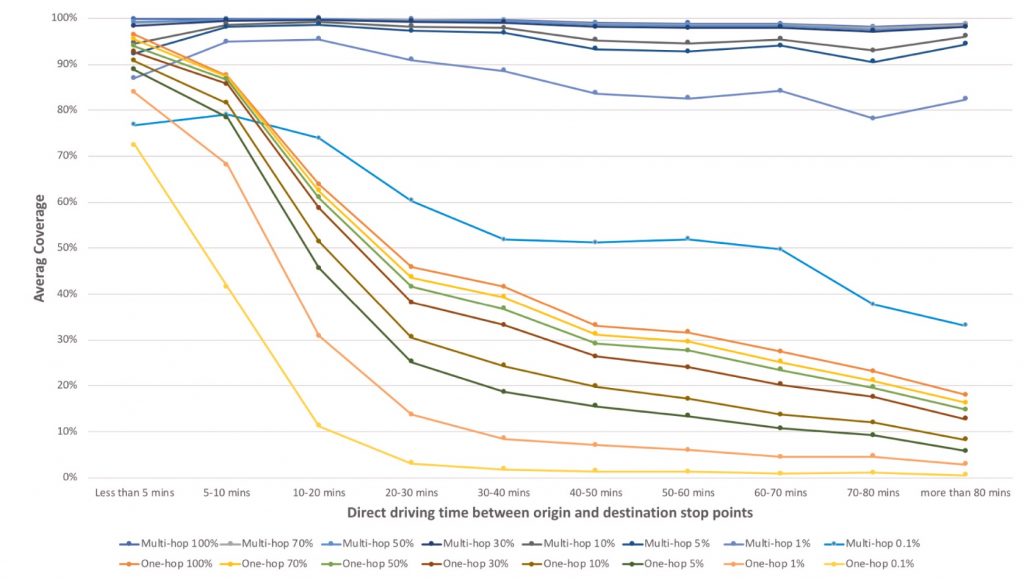
Average coverages of origin and destination stop points in the multi-hop and one-hop architectures within different sampling rates divided by the direct driving time between the stop points.
Comments are closed.