I am happy to announce that we have published a new paper: “Analyzing large-scale human mobility data: a survey of machine learning methods and applications”. In a collaboration with Boaz Lerner and Eyal Ben-Zion from Ben Gurion University and with Irad Ben-Gal from Tel Aviv University’s Industrial Engineering, we had surveyed the types of machine learning techniques used in analyzing large-scale location traces datasets and the applications that are built on them. The paper was published in the Knowledge and Information Systems Journal.
You can find the article here on the website of the journal, or download it here directly.
Abstract: Human mobility patterns reflect many aspects of life, from the global spread of infectious diseases to urban planning and daily commute patterns. In recent years, the prevalence of positioning methods and technologies, such as the global positioning system, cellular radio tower geo-positioning, and WiFi positioning systems, has driven efforts to collect human mobility data and to mine patterns of interest within these data in order to promote the development of location-based services and applications. The efforts to mine significant patterns within large-scale, high-dimensional mobility data have solicited use of advanced analysis techniques, usually based on machine learning methods, and therefore, in this paper, we survey and assess different approaches and models that analyze and learn human mobility patterns using mainly machine learning methods. We categorize these approaches and models in a taxonomy based on their positioning characteristics, the scale of analysis, the properties of the modeling approach, and the class of applications they can serve. We find that these applications can be categorized into three classes: user modeling, place modeling, and trajectory modeling, each class with its characteristics. Finally, we analyze the short-term trends and future challenges of human mobility analysis.
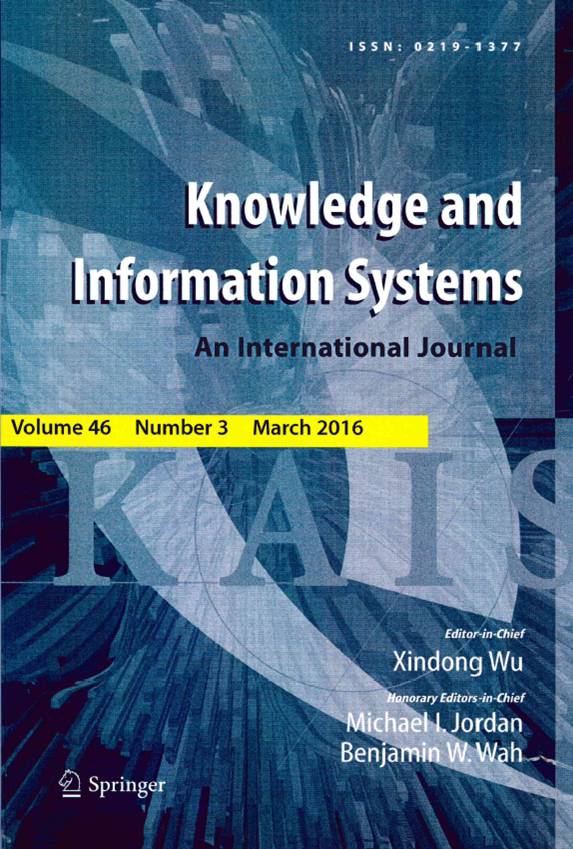
Comments are closed.